Is cyber surveillance the new mental health care?
Earlier this year Google started promoting depression tests to users in the US. Now, stateside researchers have found evidence that machine learning techniques can spot signs of depression from your Twitter feed. Will algorithms ever replace psychiatrists, or should be more excited by their other applications?
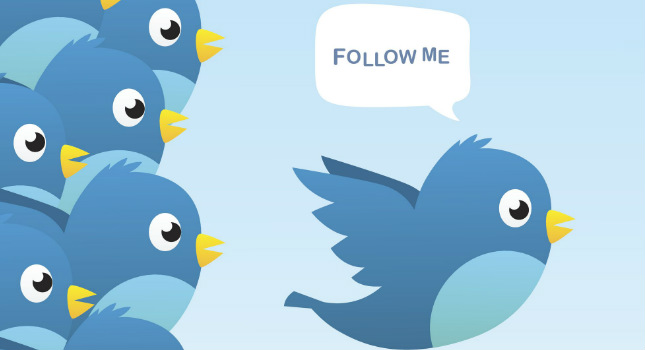
Machine learning and Artificial Intelligence (AI) are increasingly being touted as answers to our under-resourced and overburdened health care services. The use of data to shape treatment and diagnosis has potential to change the ways in which mental health care, treatment and support is provided, but this is not without its challenges.
"While we are obsessed with trying to find cast-iron machine powered ways to diagnose conditions, the actual benefits of machine learning will probably be in the realm of treatment and support."
This month Healthcare Analytics News reported a team of researchers from the University of Vermont, Stanford and Harvard found it was possible to detect depression and post traumatic stress disorder (PTSD) from people’s Twitter feeds, and to detect this before an official diagnosis was made.
The study recruited participants via Mechanical Turk, a ‘crowdwork’ platform where people sell their time or expertise completing tasks. They looked at differences between ‘healthy’ tweets and those of tweeters who were identified as having either depression or PTSD. They found that pre-created algorithms could (mostly) correctly identify participants who had diagnosis of depression or PTSD and could predict fairly well which participants would receive a diagnosis from the patterns of their language and frequency of tweeting.
Have you consent?
This issues in this study are a microcosm of those inherent in the wider discussion of machine learning and AI in mental health care. “The most obvious danger is these tools being used to speculatively diagnose people in totally inappropriate contexts, says Lydia Nicholas, digital anthropologist working in health and technologies. "The fact that the system works on public, informal conversations is more concerning here- you could be diagnosed and targeted without your consent or knowledge.”
While we are obsessed with trying to find cast-iron machine powered ways to diagnose conditions; the actual benefits of machine learning will probably be in the realm of treatment and support. Machine learning is all about pattern recognition. You feed data into a program and the program spots patterns. Where machine learning and artificial intelligence really come into their own is when they are embedded in decision systems where the patterns they spot are then used to guide action and where the result is fed back, modifying the rules for making those decisions.
"Machine learning and AI must solve an existing problem in a way that benefits those who require help or support to be useful in mental health care. The aim must be to create and validate algorithms that make guesses about what is most useful to happen at particular decision points and then trigger some form of action."
Trying to ‘spot things’ in patterns of data is risky where the feedback loop is not closed. The effects of incorrect diagnosis may take months or years to become clear. When dealing with photos it is easy to see where algorithms get things wrong; in people’s lives, less so. In August, Wired reported on a paper that found algorithms trained using existing photos tended to give answers that reflected overall prejudices; citing the example of an image recognition algorithm that tended to label people pictured in kitchens as ‘women’ and Google’s own photo recognition neural network that managed to label an African American couple ‘gorillas’.
Choosing the intervention
Machine learning and AI must solve an existing problem in a way that benefits those who require help or support to be useful in mental health care. The aim must be to create and validate algorithms that make guesses about what is most useful to happen at particular decision points and then trigger some form of action. The obvious application of such work would be the development of more useful programs to interact with people. Algorithms and machine learning could be used for diagnostic purposes, or to understand which interventions to trigger at the best times or in the most fitting context.
For example, an app could use smartphone data to learn an individual’s behaviour over time then detect when they were nearing an anxiety attack and provide encouragement or support based on algorithms for the most effective intervention. Interest is growing in the development and use of these ‘just-in-time momentary ecological interventions’.
A hypothetical personalised mental health app might learn to recognise the level of emotion someone was experiencing by sampling periodically the volume and tone of their voice. But it might also know from GPS data and time of day that the person was likely driving their kids to school. Usually the app would send a push notification to ask if everything was OK and suggest some breathing exercises or distraction activities that the person had agreed were useful. Instead, the app knows to wait and then send a message asking if the drive had been stressful, triggering a chatbot to get more information and to suggest ways to combat road rage or to make a reminder to discuss why driving the kids to school was so stress the next time they saw their therapist. In this case the app would have been trained on other people's data while also learning from data created by the user, both combining to personalise the intervention to time, place and situation.
Researcher Zach Cohen is working on a Personalised Advantage Index, which takes data from trials and research in mental health and attempts to build a picture of which treatments work for exactly which people, creating predictive algorithms to help prescribing. These algorithms are being tested against existing outcomes data to see whether the predictions they make match with the actual results that were found in real-life treatments prescribed to people.
Personal assistants such as Apple’s Siri, Amazon’s Alexa or Google’s Assistant are already utilising models where knowledge of what individuals ask; how they ask it and the context of their request shape better, more intelligent-seeming, responses. Digital Health Futurist Maneesh Juneja, wrote a recent blog post exploring the use of electronic personal assistants and chatbots around mental health. Residents of the London boroughs of Barnet, Camden, Enfield, Haringey and Islington can use a new chatbot app, NHS 111 for health advice. Trialling it, Juneja found: “Without having to wait for a human being to pick up my call, I got the advice I needed and most importantly I had time to think when answering. The process of answering the questions that the app asked was under my control. I could go as fast or as slowly as I wanted, the app wasn't trying to rush me through the questions.”
“We have to remember that research studies are essentially experiments, some fail, and others succeed," adds Juneja. "Given the complexity of biomedical research, it could be 10 years before a machine is accurately capable of determining your risk of depression from your tweets, or maybe it might never be possible, and rest as simply as a failed computation in the realm of academia."
"It's critical we look at the benefits and the risks of such an approach and co-create any new computational methods with patients as partners. Ultimately, even if we can detect depression much earlier in someone's life, are we going to be able to intervene given the difficulties for many in getting timely access to mental health care?”
While machine diagnosis might grab the headlines, it’s what machines can do in the lives of people that holds the real opportunities.
Mark Brown writes extensively on mental health and is the former editor of 1 in 4 magazine, created for and by people with mental health needs. @markoneinfour
Show your support for what you’ve read today. Enable us to keep finding and sharing the ideas that will better shape tomorrow’s mental health care.
Comments
Write a Comment
Comment Submitted